Predictive analytics leverages historical data patterns and machine learning algorithms to identify potential fraud before it occurs. Advanced systems analyze transaction streams in real-time, establishing behavioral benchmarks and flagging anomalies through dynamic risk scoring models. Neural networks detect subtle irregularities while continuous monitoring adapts to emerging fraud schemes. This proactive approach enables financial institutions to intercept suspicious activities before losses occur. The evolution of these sophisticated detection methods reveals increasingly powerful protective capabilities.
Key Takeaways
- Predictive analytics analyzes historical data patterns to establish baseline behaviors and flag deviations that could indicate potential fraud.
- Machine learning algorithms continuously process real-time transaction data to identify suspicious patterns before fraudulent activities complete.
- Dynamic scoring models instantly evaluate risk levels for each transaction by comparing them against established behavioral benchmarks.
- Neural networks detect subtle anomalies in user behavior that traditional rule-based systems might miss, preventing sophisticated fraud attempts.
- Adaptive learning systems automatically update fraud detection models as new patterns emerge, staying ahead of evolving criminal tactics.
Understanding the Evolution of Modern Fraud Detection
As financial crimes have grown increasingly sophisticated in the digital age, fraud detection systems have undergone a fundamental transformation from static rule-based approaches to dynamic predictive analytics platforms.
Modern fraud detection now leverages machine learning and advanced algorithms to identify suspicious activity across multiple data sources in real-time. This evolution reflects the necessity to combat evolving fraud patterns, particularly the emergence of sophisticated threats like synthetic identities and deep fakes.
Financial institutions have adopted proactive risk management strategies through predictive analytics, enabling continuous learning and adaptation of detection models. These systems analyze vast datasets to discern subtle patterns that might indicate fraudulent behavior, greatly improving accuracy while reducing false positives.
The implementation of real-time fraud detection capabilities allows organizations to respond to threats before they materialize into substantial losses.
The Power of Data-Driven Pattern Recognition
Data-driven pattern recognition forms the cornerstone of modern predictive analytics in fraud detection, enabling financial institutions to identify and prevent fraudulent activities with unprecedented accuracy.
Through sophisticated machine learning algorithms, these systems analyze vast quantities of historical data to establish baseline behavior patterns.
Advanced algorithms process enormous data volumes to create behavioral benchmarks, enabling precise detection of irregular patterns.
Key components of pattern recognition in fraud detection include:
- Real-time data processing capabilities that enable immediate response to suspicious patterns
- Anomaly detection mechanisms that flag deviations from established user behaviors
- Continuous learning systems that evolve with new data inputs
The integration of diverse data sources enhances the analytical capabilities of predictive models, allowing financial institutions to detect potential fraudulent activities before they materialize.
This systematic approach to pattern recognition leverages both historical and real-time transaction data, creating a robust framework for proactive fraud prevention.
Real-Time Anomaly Detection and Risk Scoring
Behavior pattern analysis systems form the foundation of modern fraud detection by continuously monitoring and evaluating transaction data against established baseline behaviors.
Dynamic scoring models process these behavioral patterns in real-time, generating risk assessments that reflect the likelihood of fraudulent activity based on current and historical data points.
The implementation of these scoring models enables financial institutions to automatically flag suspicious transactions and execute appropriate response protocols, markedly reducing the window of vulnerability for potential fraud attempts. Additionally, real-time monitoring can significantly enhance the detection of unusual patterns, allowing for quicker intervention against fraud.
Behavior Pattern Analysis Systems
Through advanced machine learning algorithms, behavior pattern analysis systems represent a critical component in modern fraud detection frameworks, enabling real-time monitoring and risk assessment of user activities.
These systems employ sophisticated data mining techniques to analyze historical behavior and identify potential fraud patterns that traditional methods might overlook.
Key capabilities include:
- Continuous learning mechanisms that adapt to emerging fraud tactics
- Risk scoring algorithms that prioritize high-risk transactions
- Real-time anomaly detection that greatly reduces false positives
Dynamic Scoring Model Implementation
Within modern fraud detection frameworks, dynamic scoring models represent a sophisticated advancement in real-time risk assessment capabilities. Through real-time data analysis and advanced machine learning algorithms, these systems continuously evaluate transactions against established behavioral patterns, enabling immediate anomaly detection and fraud identification.
The integration of diverse data sources enhances the precision of fraud detection by incorporating thorough user behavior analytics and transaction histories. This multilayered approach greatly reduces false positives while maintaining high detection accuracy.
Dynamic scoring models adapt continuously to emerging fraud tactics through automated learning mechanisms, guaranteeing sustained effectiveness against evolving threats. The implementation of proactive fraud detection capabilities allows financial institutions to intercept suspicious activities before losses occur, while continuous monitoring ensures the system remains calibrated to current risk patterns.
Machine Learning Algorithms in Predictive Fraud Analysis
Modern machine learning algorithms serve as powerful tools in the battle against financial fraud by analyzing massive datasets to identify suspicious patterns and anomalies. Through predictive analytics, these systems enable financial institutions to implement proactive detection strategies before fraudulent activities occur.
Key components of machine learning-based fraud detection:
- Supervised learning models trained on labeled data to recognize established fraud patterns
- Neural networks that detect subtle anomalies traditional methods might miss
- Continuous learning capabilities that adapt to emerging fraud schemes
The integration of advanced machine learning algorithms has revolutionized fraud detection by considerably reducing false positives while maintaining high accuracy.
This sophisticated approach allows financial institutions to process vast amounts of transactions efficiently, utilizing both supervised and unsupervised learning methods to identify patterns and suspicious activities in real-time.
Building Comprehensive Fraud Prevention Systems
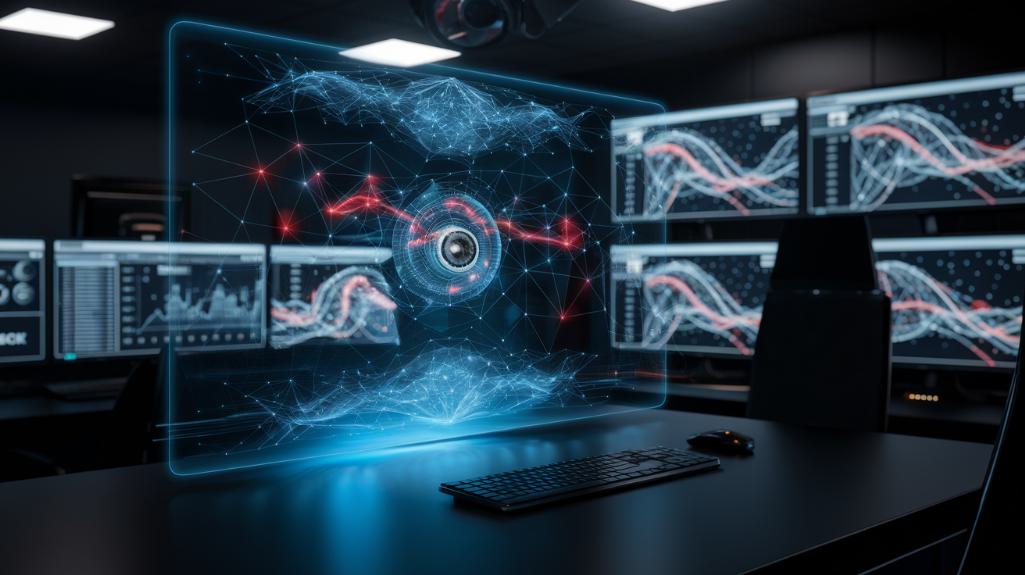
Successfully building thorough fraud prevention systems requires a strategic integration of multiple components, including advanced analytics, robust data infrastructure, and cross-organizational collaboration. These systems leverage predictive analytics and machine learning models to analyze transaction histories and behavioral data, identifying potential fraud through anomaly detection. Real-time analytics capabilities enable immediate responses to suspicious activities, while continuous model training guarantees adaptation to emerging threats.
Component | Function | Benefit |
---|---|---|
Data Quality | Guarantees accurate inputs | Reduces false positives |
Machine Learning | Analyzes patterns | Predicts fraud attempts |
Real-time Analytics | Monitors transactions | Enables rapid response |
Cross-org Collaboration | Shares insights | Strengthens detection |
The effectiveness of fraud prevention systems depends heavily on maintaining high-quality data sources and regularly updating detection algorithms to address evolving fraudulent tactics while minimizing false positives.
Transforming Customer Security Through Early Warning Signals
Early warning signals powered by predictive analytics have revolutionized customer security frameworks by detecting potential fraud before considerable damage occurs. Through advanced machine learning algorithms, financial institutions analyze behavioral anomalies and suspicious patterns in real-time, enabling proactive risk mitigation.
Key components of early warning detection include:
- Real-time monitoring of transaction patterns and device access
- Natural language processing for identifying manipulative communications
- Continuous adaptation to emerging fraud schemes
The system’s ability to process vast amounts of historical and current data transforms traditional fraud detection approaches.
Future-Proofing Fraud Detection With Advanced Analytics
Advanced analytics platforms employ real-time pattern recognition to detect and prevent fraudulent activities through continuous monitoring of transaction streams and behavioral markers.
Machine learning models adapt to emerging fraud schemes by analyzing historical data patterns and incorporating new threat indicators into their detection algorithms.
This combination of real-time monitoring and adaptive learning creates a dynamic defense system that evolves alongside sophisticated fraud techniques, enabling financial institutions to maintain robust security measures against future threats.
Real-Time Pattern Recognition
Real-time pattern recognition represents a critical advancement in fraud detection systems, leveraging sophisticated machine learning algorithms to analyze transactions at the moment they occur.
This proactive approach enables financial institutions to identify anomalies instantly, preventing potential losses before they materialize.
Key capabilities of real-time pattern recognition include:
- Continuous learning from historical data to adapt to emerging fraud techniques
- Simultaneous processing of vast transaction data through big data integration
- Immediate flagging of atypical behaviors and suspicious patterns
Adaptive Learning Models
The evolution of adaptive learning models marks a transformative shift in fraud detection capabilities, enabling financial institutions to stay ahead of increasingly sophisticated criminal tactics.
Through advanced predictive analytics and machine learning algorithms, these models continuously refine their fraud detection mechanisms by processing new data points and identifying emerging threats.
The integration of real-time data analysis with anomaly detection and behavioral analysis allows these systems to instantly flag suspicious activities.
Big data technologies enhance the scalability of these models, ensuring efficient processing of massive transaction volumes.
Continuous model training optimizes performance by adapting to new fraud patterns while minimizing false positive rates.
This systematic approach to fraud detection creates a robust defense mechanism that evolves alongside criminal methodologies, providing financial institutions with proactive protection against emerging threats.
Frequently Asked Questions
How Is Data Analytics Used to Detect Fraud?
Data analytics employs machine learning and data mining to perform transaction analysis, anomaly detection, and behavioral profiling, utilizing historical data for pattern recognition and predictive modeling in extensive fraud detection and risk assessment.
How Predictive Analytics Strive to Tell What Is Likely to Happen?
Predictive modeling techniques leverage historical data analysis and machine learning applications to analyze behavior prediction patterns, while anomaly detection algorithms and real-time monitoring systems enable statistical forecasting of future scenarios and trends.
How Does AI Identify Fraud?
AI employs machine learning algorithms for fraud detection through real-time analysis, transaction monitoring, and pattern recognition. Systems perform data mining, behavioral analysis, and anomaly detection to generate automated risk assessment alerts.
What Is the Most Common Method of Early Fraud Detection?
Transaction monitoring systems and machine learning algorithms analyze behavioral patterns and transaction anomalies, leveraging historical data analysis to detect unusual activities that deviate from established user patterns through automated alerts systems.
Conclusion
Predictive analytics continues to revolutionize fraud detection through advanced machine learning algorithms, real-time pattern recognition, and thorough risk scoring systems. By leveraging vast datasets and automated anomaly detection, organizations can identify potential fraud before monetary losses occur. As computational capabilities expand and analytical models become more sophisticated, predictive systems will remain critical in maintaining financial security and preventing emerging fraud schemes.